MRL 2023 Shared Task on Multi-lingual Multi-task Information Retrieval
Description and Objectives
Large language models continue to demonstrate outstanding performance in many applications that require competence in language understanding and generation. This performance is especially prominent in English, where large amounts of public evaluation benchmarks for various downstream tasks are available. However, the extent to which language models can be reliably deployed in terms of different languages and domains are not still well established. Recent efforts in creating benchmarks, such as the XTREME-UP benchmark, providing extended ranges of languages in large sets of downstream tasks allow a more inclusive setting for studying different characteristics of large language models under different categories and properties of data and domains. In this new shared task we extend the XTREME-UP benchmark to include various out-of-domain data sets in a selected set of data-scarce and typologically-diverse languages. Our evaluation benchmark consists of the multi-domain public data sets in XTREME-UP, where we provide a multi-task evaluation setting, in particular in the tasks of Named Entity Recognition (NER) and Reading Comprehension (RC), on test sets curated from articles on Wikipedia. The main objective of the shared task is to assess and understand the multilingual characteristics of the inference capability of multilingual language models in understanding and generating language based on logical, factual or causal relationships between knowledge contained over long contexts of text, especially under low-resource settings.
Tasks and Evaluation
With the advancement of language models accessing and processing tons of information in different formats and languages, it has become of great importance to be able to assess the capabilities to access and provide the right information useful to different audiences. In this shared task, we provide a multi-task evaluation format that assesses information retrieval capabilities of language models in terms of two subtasks: named entity recognition and question answering.
Named Entity Recognition (NER) is a classification task that identifies phrases in a text that refer to entities or predefined categories (such as dates, person, organization and location names) and it is an important capability for information access systems that perform entity look-ups for knowledge verification, spell-checking or localization applications. The XTREME-UP dataset contains processed data from MasakhaNER (Adelani et al., 2021) and MasakhaNER 2.0 (Adelani et al., 2022) in the following languages: Amharic, Ghomálá, Bambara, Ewe, Hausa, Igbo, (Lu)Ganda, (Dho)Luo, Mossi (Mooré), Nyanja (Chichewa), Nigerian Pidgin, Kinyarwanda, Shona, Swahili, Tswana (Setswana), Twi, Wolof, Xhosa, Yoruba and Zulu. The objective of the system is to tag the named entities in a given text, either as a person (PER), organization (ORG), or location (LOC) (Our tag set uses $$ as delimiter).
Question answering (QA) is an important capability that enables responding to natural language questions with answers found in text. Here we focus on the information-seeking scenario where questions can be asked without knowing the answer—it is the system’s job to locate a suitable answer passage (if any). The information-seeking question-answer pairs tend to exhibit less lexical and morphosyntactic overlap between the question and answer since they are written separately, which is a more suitable setting to evaluate typologically-diverse languages. Here, the system is given a question, title, and a passage and must provide the answer—if any—or otherwise return that the question has “no answer” in the passage. The XTREME-UP benchmark currently contains QA data only in Indonesian, Bengali, Swahili and Telugu. The competing systems will therefore be required to infer information from different language annotations.
Evaluation in the generative task will use character error rate (CER) and character n-gram F-score rather than their word-level counterparts as they enable more fine-grained evaluation and are better suited to morphologically-rich languages. We obtain a final score by averaging the scores of QA and NER, evaluated with F1 accuracy.
Data and Languages
The training and validation data sets that can be used for building multi-task information retrieval systems are directly accessible on the XTREME-UP repository. The test sets for official evaluation will be released ten days before the submission date, and will be in the following languages: Igbo, Indonesian, Swiss German, Turkish, Uzbek, Yoruba. We also anticipate that there will be one or two surprise languages in the final test sets.
Participation
Interested parties are invited to contact mrlw2023@gmail.com or join the google group mrl-shared-task-2023@googlegroups.com to be involved in the competition.
All participating systems will be evaluated together with our baselines against the same held-out test set, to be released shortly before evaluation. Submitted systems can compete in some or all sub-tasks.
Participating teams will be invited to submit a short paper describing their work to the MRL workshop and to present it in a special session in the workshop. Paper submissions must follow the EMNLP paper format and sent to Softconf Conference Link of MRL 2023 before the paper submission deadline.
Important dates
September 18, 2023: Release of testing data
September 27, 2023: Deadline to release external data and resources used in systems
September 28, 2023: Deadline for submission of systems
October 9, 2023: Release of rankings and results
October 14, 2023: Deadline for submitting system description papers
October 16, 2023: Paper notifications
October 21, 2023: Camera-ready papers and posters due
December 7, 2023: Workshop
Ranking
The systems will be evaluated based on the global ranking on all benchmark languages. Participants can submit systems that are language-specific (monolingual) and their systems will be evaluated as a partial submission to the specific language their system is trained on.
Additional resources
The shared task allows participants to use external resources or tools as long as they are openly available and can be, in theory, used by other participants for research purposes. In case participants decide to use external resources and data in their system they should contact the organizers in case the specific resources would be permitted, in such cases specific information on the used resources and how they can be obtained should be shared via email by September 15th, 2023.
Organizers
David Adelani, UCL and Google Deepmind
Duygu Ataman, New York University
Chris Emezue, TU Munich and Mila
Omer Goldman, Bar Ilan University
Mammad Hajili, Microsoft
Sebastian Ruder, Google Deepmind
Francesco Tinner, University of Amsterdam
Genta Indra Winata, Bloomberg
Shared Task Prize
The winning team will receive an award of 500 USD and will be given a presentation during the workshop.
Sponsors
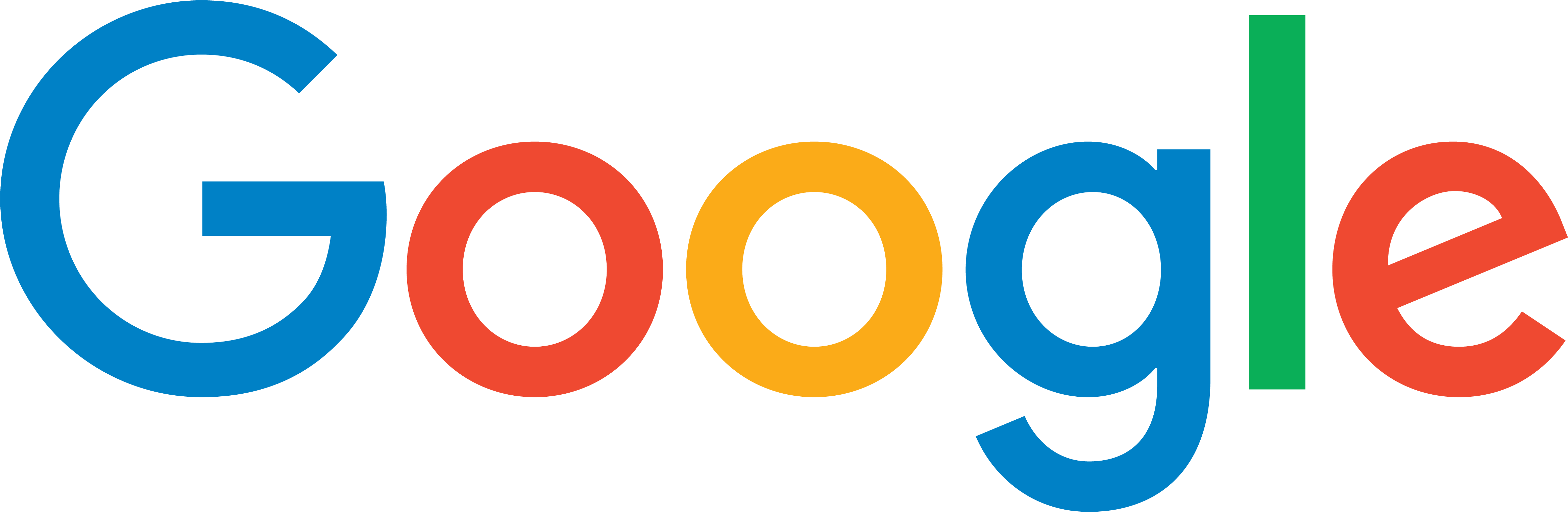
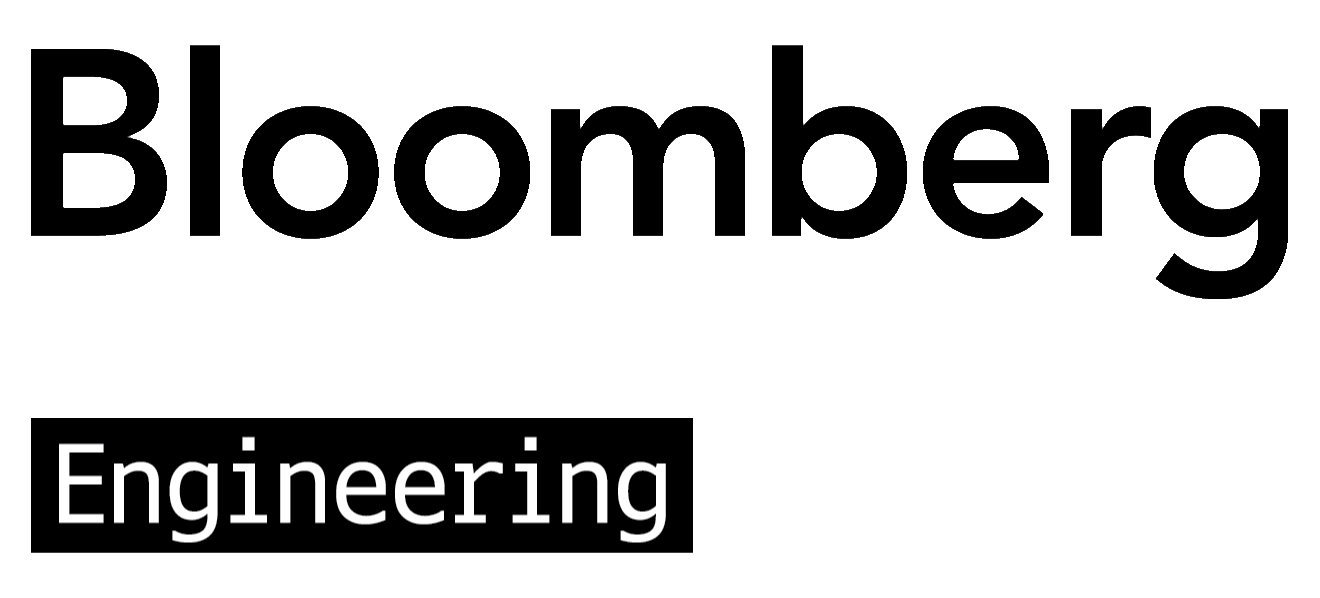
Interested in being a Sponsor? Contact us!